The synthetic intelligence (AI) panorama continues to evolve, demanding fashions able to dealing with huge datasets and delivering exact insights. Fulfilling these wants, researchers at NVIDIA and MIT have lately launched a Visible Language Mannequin (VLM), VILA. This new AI mannequin stands out for its distinctive capability to motive amongst a number of photographs. Furthermore, it facilitates in-context studying and comprehends movies, marking a major development in multimodal AI methods.
Also Learn: Insights from NVIDIAβs GTC Convention 2024
The Evolution of AI Fashions
Within the dynamic area of AI analysis, the pursuit of steady studying and adaptation stays paramount. The problem of catastrophic forgetting, whereby fashions battle to retain prior information whereas studying new duties, has spurred progressive options. Methods like Elastic Weight Consolidation (EWC) and Expertise Replay have been pivotal in mitigating this problem. Moreover, modular neural community architectures and meta-learning approaches supply distinctive avenues for enhancing adaptability and effectivity.
Also Learn: Reka Reveals Core β A Chopping-Edge Multimodal Language Mannequin
The Emergence of VILA
Researchers at NVIDIA and MIT have unveiled VILA, a novel visible language mannequin designed to deal with the restrictions of current AI fashions. VILAβs distinctive strategy emphasizes efficient embedding alignment and dynamic neural community architectures. Leveraging a mix of interleaved corpora and joint supervised fine-tuning, VILA enhances each visible and textual studying capabilities. This manner, it ensures sturdy efficiency throughout numerous duties.
Enhancing Visible and Textual Alignment
To optimize visible and textual alignment, the researchers employed a complete pre-training framework, using large-scale datasets akin to Coyo-700m. The builders have examined numerous pre-training methods and integrated strategies like Visible Instruction Tuning into the mannequin. Consequently, VILA demonstrates outstanding accuracy enhancements in visible question-answering duties.
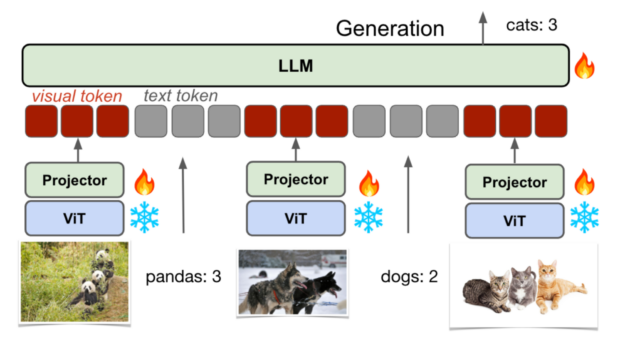
Efficiency and Adaptability
VILAβs efficiency metrics converse volumes, showcasing important accuracy positive aspects in benchmarks like OKVQA and TextVQA. Notably, VILA reveals distinctive information retention, retaining as much as 90% of beforehand discovered data whereas adapting to new duties. This discount in catastrophic forgetting underscores VILAβs adaptability and effectivity in dealing with evolving AI challenges.
Also Learn: Grok-1.5V: Setting New Requirements in AI with Multimodal Integration
Our Say
VILAβs introduction marks a major development in multimodal AI, providing a promising framework for visible language mannequin growth. Its progressive strategy to pre-training and alignment highlights the significance of holistic mannequin design in reaching superior efficiency throughout numerous purposes. As AI continues to permeate numerous sectors, VILAβs capabilities promise to drive transformative improvements. It’s absolutely paving the way in which for extra environment friendly and adaptable AI methods.
Observe us onΒ Google InformationΒ to remain up to date with the most recent improvements on the earth of AI, Information Science, &Β GenAI.